Introduction
In the realm of convalescence, the advent of knee walkers has brought forth a revolution, presenting a complaisant and expedient alternative to the age-old crutches of yore. These novel contrivances offer a firm and secure mode of conveyance, thereby assisting convalescents in the swift restoration of autonomy and mobility during the convalescent period.
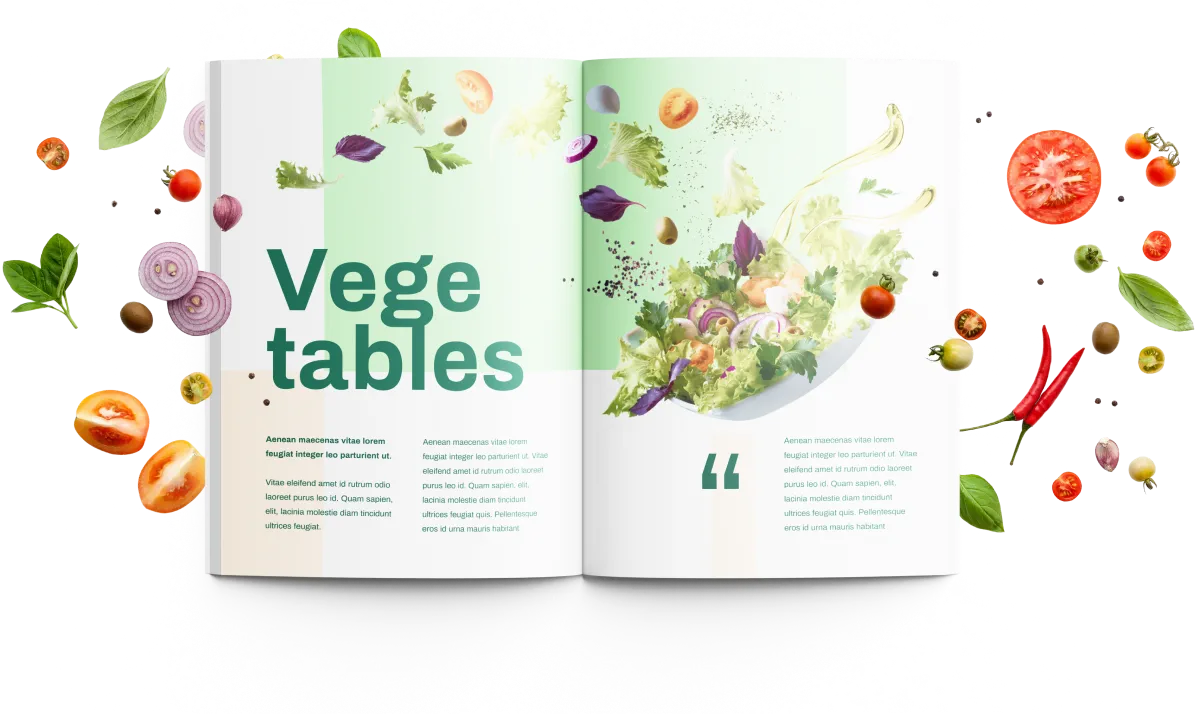
The Evolution of Knee Walkers
The chronicles of knee walkers narrate a tale of metamorphosis through the annals of bygone eras, metamorphosing from rudimentary prototypes to sophisticated and feature-laden ambulatory auxiliaries. In ancient times, when reliance on traditional crutches was endemic, advancements in technology prompted the yearning for more efficient and user-friendly apparatus, thus engendering the birth of contemporary knee walkers.
The antecedent iterations of knee walkers oft manifested as unwieldy and arduous to operate, constraining the mobility and comfort of the user. As sagacity dawned upon manufacturers, acknowledging inherent deficiencies in these nascent designs, they began integrating ergonomic functionalities, lightweight materials, and adaptable components to enrich the user's experience.
How Knee Walkers Enhance Rehabilitation
The utilization of knee walkers confers a boon upon the process of convalescence by alleviating strain on the superior anatomy and endorsing proper posture. Unlike crutches, notorious for inducing discomfort and languor, knee walkers proffer a semblance of naturalism and ergonomics in locomotion whilst convalescing from infirmities or surgical procedures.
By furnishing sustenance and poise to the limb in convalescence, knee walkers facilitate the equitable distribution of mass, mitigating strain on articulations and musculature. This expedites convalescence and forestalls collateral injuries that could arise from improper load-bearing during the period of recuperation.
Moreover, knee walkers empower users to uphold an active lifestyle during convalescence, enabling them to partake in diurnal pursuits with enhanced facility and aplomb. Whether traversing interiors or exteriors, these instruments propose versatility and maneuverability, facilitating navigation across diverse milieus with ease.
Tips for Using a Knee Walker Effectively
To optimize the dividends of a knee walker, judicious application and upkeep are imperative. Ensuring the elevation of the knee platform is conducive to positioning the languid limb comfortably and securely is paramount. Additionally, the routine inspection of the brakes, wheels, and framework is indispensable to guarantee safe and seamless operation.
Whilst employing a knee walker, it behooves one to adopt measured strides and moderate momentum to preserve equilibrium and poise. Eschewing abrupt motions or precipitate turns that may precipitate mishaps or stumble is prudent. Through compliance with these precepts and the interspersion of periodic intervals for respite and adjustment, individuals shall derive maximal benefit from the tenure with their knee walkers, thus optimizing their journey of recuperation.